Does AI Have a Role in Discharge Instructions? Exploring the Clinical Utility of AI-Generated Discharge Instructions Following Interventional Spine Procedures
Cite as: Glicksman M, Solanki P, Brancolini S, et al. Does AI have a role in discharge instructions? exploring the clinical utility of AI-generated discharge instructions following interventional spine procedures. ASRA Pain Medicine News 2024;49. https://doi.org/10.52211/asra050124.010.
Existing literature indicates that up to one-third of Americans have low health literacy, and 40 percent of patients do not fully understand their diagnosis post-discharge.1,2 In addition, patients are often unaware of their own limitations in comprehension, which leads to treatment, medication, and activity non-adherence.1 These problems may be amplified in patients receiving treatment for chronic pain where multimodal treatment modalities are routinely employed, and multiple diagnoses can simultaneously contribute to morbidity.
Both inpatient and outpatient discharge instructions (DI), therefore, can be an invaluable resource for patients and caregivers, and creating relevant and comprehensible DI is a critical step in preventing adverse events following direct patient care.2 The components of these instructions may vary depending on the patient setting, but typically include medication changes, interventions, activity limitations, follow-up appointments, contact information, and self-monitoring guidelines.1,3 Research indicates that clear DI may improve patient satisfaction, which is fundamental to the patient-physician relationship.1 They may also assist in improving patient understanding of medicine and overall health literacy, translating to better patient care.1 This improved patient health literacy and satisfaction has significant financial implications, not only in reducing overall healthcare costs, but also in minimizing potentially preventable readmissions and increasing Hospital Consumer Assessment of Healthcare Providers and Systems scores—two key components of value-based reimbursement under current health policy guidelines.1,2,4
Unfortunately, prior analyses of DI reveal that critical errors are common. This includes medication dosage and duration errors as well as an alarming rate of incomplete instructions.1 Variations in physician expertise and available time to dedicate to the discharge process are two factors that contribute to DI discrepancies.2 To address these discrepancies, standardized DI may be created. However, widely applying standardized DI may fail to address specific comorbidities or medical considerations for individual patients. Therefore, it is paramount to create DI that correctly and thoroughly address each patient’s past and future care. Given the variety and medical complexity of patients with chronic pain, developing a method to generate DI that are both accurate and individualized could be particularly beneficial in this population. Due to the continually expanding capabilities of artificial intelligence (AI) within medicine, this technology holds the potential to help create such instructions.
Since the 1970s, the capabilities and roles of AI have drastically evolved. It now offers the ability to function within multiple areas of medicine—from basic biomedical and translational research to clinical practice and beyond.5 While research in AI has largely focused on the intricacies of AI and the current/potential applications that this technology may have for clinical practice, there is a notable scarcity of literature addressing how AI may assist with the discharge process. Therefore, we sought to briefly explore the potential benefits and limitations of utilizing this technology to create personalized DI for patients who receive multimodal care from a pain management physician. In addition, we performed a brief pilot experiment to explore the capabilities of AI to function in this setting.
Potential Benefits of AI in the Creation of DI
One significant benefit of AI is in its potential to generate comprehensible DI and thus to improve patient health literacy. In a prior study evaluating the ability of an AI-based system to improve hospital DI for heart failure, the authors demonstrated that after briefly training AI to create an algorithm, there was a statistically significant improvement in the readability of DI achieved by lowering the requisite reading level.4 This holds significant implications given that some reports suggest that mean reading levels of patients may range from grades three to ten depending on the hospital setting.1 Thus, by simplifying and tailoring discharge instructions for chronic pain to a particular literacy level, this alone may dramatically improve the patient’s understanding, engagement, and satisfaction with his or her multimodal care plan.
Another significant benefit of AI originates from large language models (LLMs) that are incorporated into many available AI technologies. These models are trained on large amounts of text data to generate human-like answers with high degrees of accuracy.6,7 As has been suggested, these LLMs may be utilized to personalize learning experiences.6 Thus, this modality may be able to function similarly in integrating patient information into the creation of individualized DI. As compared to human-generated DI, one could anticipate that these tools may provide far greater personalization while also conferring a time savings benefit for physicians. Their standardized approach may further reduce traditional errors in DI as previously described.
Simplifying and tailoring discharge instructions for chronic pain to a particular literacy level, this alone may dramatically improve the patient’s understanding, engagement, and satisfaction with his or her multimodal care plan.
Utilizing AI to Generate DI Following Interventional Spine Procedures: A Pilot Experiment
One example of a popular and publicly available LLM is Chat Generative Pre-trained Transformer 3.5 (ChatGPT-3.5) operated by OpenAI. To briefly examine the ability of AI to generate DI, we prompted this model to create DI tailored to the chronic pain patient population. We incorporated common comorbidities (eg, diabetes mellitus, hypertension), clinical events (eg, lumbar epidural steroid injection at L5-S1), and history of procedural complications (eg, post-dural puncture headache). To create each set of DI, we provided ChatGPT-3.5 with the following prompts: “Can you write discharge instructions for a patient seen in a chronic pain clinic who just had a lumbar epidural steroid injection at L5-S1, specifically for a patient with a history of [(A) diabetes mellitus, (B) hypertension, or (C) post-dural puncture headache]?” The output of the first prompt for diabetes mellitus is shown in Figure 1. These results were first reviewed and formatted by a board-certified pain medicine specialist.

The DI generated by ChatGPT-3.5 are encouraging. These instructions reference the relationship between the specified medical history and the recent procedure as well as providing succinct, important, and accurate information tailored to each comorbidity. For example, prompt A generated a response that included additional steps for blood sugar monitoring and diabetes management/follow-up while also reminding patients to check their glucose throughout other steps. Prompt B resulted in a similar set of DI but focused on blood pressure monitoring. Prompt C generated a particularly impressive response, noting the relationship between lumbar epidural steroid injections and post-dural puncture headaches, and incorporated additional monitoring for symptoms of this procedural complication. The results were not entirely faultless however, as noted by the overemphasized and potentially inaccurate statement regarding the relationship between NSAIDs and glycemic control.
Current Limitations of AI in the Creation of DI
Despite these encouraging results, the broad adoption and integration of AI – and thus LLMs – into the healthcare field faces several challenges. Importantly, any information obtained from the electronic health record that is necessary for information, such as patient diagnoses, medication dosages/duration, or follow-up visits, poses critical ethical issues. This includes previously described concerns regarding model biases and patient privacy.4 Moreover, the information in the chart may not be sufficient for creating safe and effective transitions of care. A prior study demonstrated that 11 percent of the information in a discharge summary is not derived from any documentation at all and requires physicians’ memories or reasoning.8 Alternatively, the patient’s chart could contain extraneous or outdated information that needlessly clutters the discharge summary. To our knowledge, there is no currently existing literature evaluating how these considerations may impact DI rather than discharge summaries.
There is also concern that LLMs may generate text that is incorrect or nonsensical when the model has limited contextual understanding due to biases or “noise” in the underlying training data.9 LLMs transform prompts and training data into an abstraction and then generate text as an extrapolation from the prompt provided. The result of this extrapolation is not necessarily supported by any training data but is the most correlated response for the given prompt.7 Thus, electronic health records alone may not yet provide enough information for these tools to generate accurate output. In our testing, the board-certified pain medicine specialist only had to make minor grammatical/formatting revisions. While encouraging, this highlights the concern that LLM-generated DI may be too complex to be understood or formatted appropriately. One recent study demonstrated that proper formatting plays a fundamental role in increasing the understandability of hospital DI.10 Although LLMs may be prompted to respond in a particular format (eg, question and answer, paragraph) or to address a certain literacy level, optimizing these elements across different healthcare settings and patient populations while simultaneously ensuring factual correctness in LLM-generated DI warrants further research.
Conclusion
Despite these limitations, LLMs represent an exciting and evolving tool to be utilized in the creation of DI following direct patient care. The objective of this brief article and preliminary pilot testing was not to validate or dismiss the ability of AI to generate DI but rather to start a conversation regarding how AI can assist in creating personalized DI within a pain medicine setting. As such, future research is required to fully evaluate the ability of this technology to generate accurate, high-quality DI. This may involve clinical trials assessing both physician and patient satisfaction/understanding of these instructions as well as evaluating future healthcare outcomes and costs. This research will provide a significant dual-benefit in providing additional training data that may refine the output accuracy, readability, and personalization of LLM-generated DI. However, because the extrapolation process of LLMs cannot yet replace human thought or decision-making, this output should first undergo considerable review and revision prior to implementation in clinical practice. Thus, while LLMs may not yet be ready to independently create DI, they may be used as a tool to improve the accuracy, readability, and personalization of DI in a cost- and time-efficient manner.




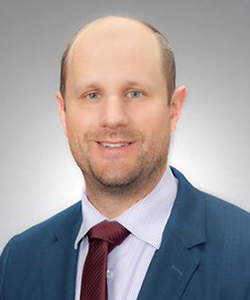
References
- DeSai C, Janowiak K, Secheli B, et al. Empowering patients: simplifying discharge instructions. BMJ Open Qual 2021;10(3):e001419. https://doi.org/10.1136/bmjoq-2021-001419
- Rodwin BA, Bilan VP, Gunderson CG, et al. Improving the quality of inpatient discharge instructions: an evaluation and implementation of best practices. South Med J 2021;114(8):445-9. https://doi.org/10.14423/SMJ.0000000000001284
- Reiter K. A look at best practices for patient education in outpatient spine surgery. AORN J2014;99(3):376-84. https://doi.org/10.1016/j.aorn.2014.01.008
- Tuan AW, Cannon N, Foley D, et al. Using machine learning to improve the readability of hospital discharge instructions for heart failure. medRxiv [Preprint]. June 20, 2023. https://doi.org/10.1101/2023.06.18.23291568
- Yu KH, Beam AL, Kohane IS. Artificial intelligence in healthcare. Nat Biomed Eng 2018;2(10):719-31. https://doi.org/10.1038/s41551-018-0305-z
- Kasneci E. et al. ChatGPT for good? On opportunities and challenges of large language models for education. Individ. Differ 2023;103:102274. https://doi.org/10.1016/j.lindif.2023.102274
- Azamfirei R, Kudchadkar SR, Fackler J. Large language models and the perils of their hallucinations. Crit Care 2023;27(1):120. https://doi.org/10.1186/s13054-023-04393-x
- Ando K, Okumura T, Komachi M, et al. Is artificial intelligence capable of generating hospital discharge summaries from inpatient records? PLOS Digit Health 2022;1(12):e0000158. https://doi.org/10.1371/journal.pdig.0000158
- Alkaissi H, McFarlane SI. Artificial hallucinations in ChatGPT: implications in scientific writing. Cureus2023;15(2):e35179. https://doi.org/7759/cureus.35179
- Chadwick W, Bassett H, Hendrickson S, et al. An improvement effort to optimize electronically generated hospital discharge instructions. Hosp Pediatr 2019;9(7):523-9. https://doi.org/1542/hpeds.2018-0251